Who should be the player of the year?
In every sport, arguments are made over who is the league’s best player, citing player statistics, team success, and how an individual plays relative to others around them. Many have attempted to combine this in an “all-in-one rating” to describe player performance or to predict future success.
Opta Analyst and our parent company Stats Perform introduced DRIP – our Daily-Updated Rating of Individual Performance – back in 2021. We continue to use it to assess player talent in the NBA. DRIP is predictive, so its utility is to measure a player’s future success in the league. Now, we’re adding a reflective metric to help answer the question we started with.
Introducing our college basketball Wins Above Replacement (WAR) – our calculation to measure a player’s actual performance over the course of a season. This metric tells us how many wins a player would add over a “replacement” player. In college basketball, the value of a “replacement” player is roughly equivalent to your average bench player on an average Division I team.
We’ll start by explaining our model, then get to its application to recent men’s and women’s NCAA basketball seasons.
Calculation
In order to arrive at an output for measuring wins above a replacement player, we combine actual box score and play-by-play data with features such as plus/minus, field goals made, assists, free throw rate, steals and more. Raw numbers are adjusted on a per 100 possessions basis.
These numbers are used to estimate the impact a player has on the court per game, then aggregated over a time period (i.e. per season). We are calling this reflective metric VAPR (Value Added Performance Rating). Like other reflective all-in-one basketball metrics, VAPR’s output can be read as a points contribution above or below the NCAA average per 100 possessions played.
Once this calculation is made, VAPR is adjusted by minutes played to get WAR. The amount of time spent on the court is a necessary step in the process of reaching a reliable WAR output as it helps contribute to the team’s overall success in a game and over a season.
VAPR is a rate-based stat; a player could play 2 minutes in a season, post positive numbers (like making both field-goal attempts in a season) and would thus have a high VAPR. Adjusting by minutes helps negate these instances as high-value players. VAPR needs a minimum number of games or minutes played to display on a leaderboard while WAR does not.
Technically speaking, how did we get there? We ran multi-layered perceptron (MLP) models and picked the best model using weighted R-squared scores. MLP models are a good approach for this calculation due to their ability to parse data that is not linearly separable, and they do a better job of preventing overfitting when compared to, say, a tree-based model. This model is also trained on over a decade of college basketball data, thus helping the accuracy and precision of the model.
A replacement-level player will have a VAPR of minus-2. Among players who played at least 500 minutes in a given season, the median VAPR is about 0.19 while the median end-of-season WAR is roughly 1.15.
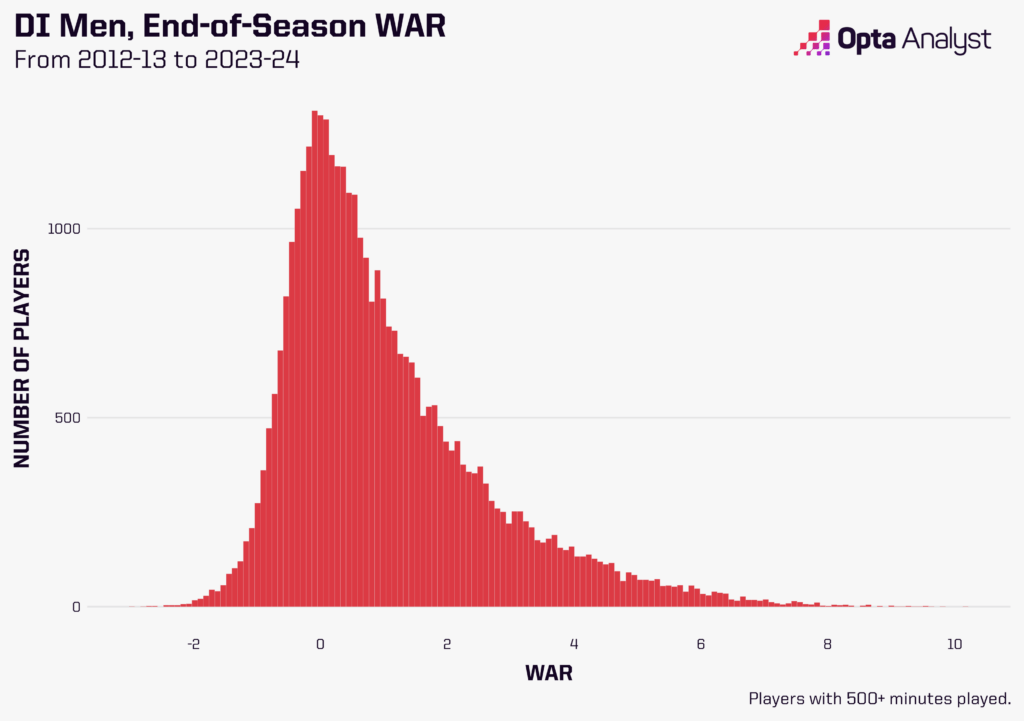
Of course, not every player gets the same number of minutes or even the same number of games due to various tournaments throughout the season. We have also added a WAR per 40 calculation, which is a players’ WAR if the team played 40 games. For example, if a player’s WAR is 5.00 and the team played 30 games, the player’s WAR per 40 would be 5.00/30*40 = 6.67.
If you’re familiar with our previous basketball work, you may be asking why we’ve gone to this trouble if we already had DRIP. Couldn’t we just create DRIP for college basketball?
The key difference is VAPR combines actual box score and play-by-play data while DRIP models each statistic for future success. DRIP is a projection of future performance, while VAPR is an evaluation of talent. WAR layers on playing time to perhaps best answer that player of the year question.
Positional and Team Components
You might recognize WAR from baseball, being one of the premier advanced metrics in the sport. In baseball, WAR breaks down players’ value by measuring how many wins they are worth relative to a replacement-level player at the same position (where a replacement-level player is the equivalent of a minor league replacement or a fill-in free agent).
The positional aspect is key, and players may differ in WAR despite having the same numbers. For example, if a second baseman and a left fielder have the same overall production (hitting, fielding, running, etc.), the second baseman would likely have a better WAR because the value of a replacement-level second baseman is lower than the value of a replacement-level left fielder (since second base is a more difficult position to play).
Positions are a factor in our men’s and women’s college basketball WAR but are organized differently. Rather than classifying players by traditional basketball positions like guard, forward or center, we classify them through numbers that better determine what position they might be. Positions in college basketball are much more arbitrary than in baseball and even in the NBA, thus a method like this better captures how a player performs relative to a replacement-level player of his caliber.
This classification clusters players by their offensive and defensive rebounds, blocks and assists into a spectrum that aligns with an expected position. This helps identify players that might be listed as one position but have a playing style like another (think Nikola Jokic or Robbie Avila). It also helps distinguish two players who are traditionally the same position but play differently (think Zach Edey versus Johni Broome). By doing this, we can compare all players’ WAR collectively instead of looking at WAR by position.
Not only are the players compared relative to a replacement-level player, but their WAR is adjusted by the level of play. In college basketball, there is a much larger disparity in talent level, so much so that it must be scaled to estimate a player’s true talent. For example, a player having a 30-point game against an AP Top 25 team is more impressive than the same player having a 30-point game against a team that will manage a few wins over the course of the season.
This is where team ratings play a big part. Our team rating metric, TRACR (Team Rating Adjusted for Conference and Roster), is a net efficiency metric that measures how good a team performs offensively and defensively relative to an average team in Division I (kind of like a replacement-level player!). TRACR adjusts after each game, rewarding teams that do well against top teams and punishing teams that perform poorly against teams they should have done well against.
Each team’s TRACR is adjusted to per-100 possessions. For example, if Team A has a TRACR of 30.0 and plays Team B that has a TRACR of 0.0, it would outscore Team B by about 0.3 points per possession. If Team A averages 70 possessions, then it would outscore Team B by 21 points on average. That is a significant spread, hence why it is important to include such a team-level component in a player calculation. Each player’s WAR is broken down game-by-game, with each game adjusted by their opponent’s TRACR entering that day.
It is also important to note that the disparity in women’s college basketball is even larger than in men’s. The undefeated 2023-24 South Carolina squad finished the season with a TRACR rating of 62.5 – 10 points higher than any other DI school. TRACR expected the Gamecocks, who averaged about 72 possessions per game, to outscore an average team by 45 points!
Thus, an opponent adjustment is critical. This is also why, on average, there are more upsets in the men’s NCAA Tournament than the women’s (but when they do happen, they are thrillers).
Leaderboards
Now for the fun part.
Let’s take a look at the top college basketball seasons in recent history to check our work.
It should not come as any surprise that almost all the players on this list took March Madness by storm and helped their team go deep in the tournament. Whether it was Trey Burke’s clutch scoring in 2013, Frank Kaminsky leading a talented Wisconsin squad over undefeated Kentucky in the Final Four, or Zach Edey’s play throughout the entire 2023-24 season en route to a runner-up performance for Purdue.
This table also illustrates how WAR encapsulates more than just scoring, otherwise the top 10 would comprise of players like Trae Young, Doug McDermott or even Chris Clemons. It is a measure of how valuable a player is in all aspects relative to a replacement-level player in Division I. WAR understands the offensive value beyond scoring, like Markquis Nowell’s 19-assist game in the Sweet 16 in 2023 or Michael Carter-Williams averaging 7.3 assists and 2.8 steals in his final season with Syracuse.
WAR incorporates features on the other side of the ball as well, such as defensive box score statistics like blocks and steals, as well as how an opponent performs while a player is on the court. Mikal Bridges, Zach Edey and Jevon Carter were excellent players offensively but were also as valuable defensively. Carter was even named the Naismith Defensive Player of the Year in 2017-18.
WAR factors in every part of a player’s game, not just one aspect.
Onto the women’s game, for which our WAR analysis will run just as deep.
Should it come as any surprise that Caitlin Clark’s last two years are on top? If they were not, we likely would have started over with our calculations.
Clark’s 13.8 WAR in 2023-24 is the highest among any DI player, men’s or women’s, between the 2012-13 and 2023-24 seasons. If Iowa, which went 34-5 and was the runner-up in the NCAA Tournament in 2023-24, had to replace Clark with a replacement-level player in Division I for the entire season, we’d expect the Hawkeyes to finish with 13 or 14 fewer wins (assuming average opponents).
Now, the Hawkeyes probably would have replaced Clark with someone above a replacement level, but they may not have made it to the championship game without her. Anyone that has watched her knows how valuable she was to Iowa and how she was better than anyone on the court – and our women’s WAR illustrates just how valuable she really was.
It should also not come as any surprise that the list is dominated by Geno Auriemma’s best. Between 2012-13 and 2023-24, 14 of the top 25 in WAR played for the UConn Huskies. UConn has a 442-33 (.925) record in those seasons, by far the best in Division I, men’s or women’s. Stewart, Mosqueda-Lewis and Faris led their teams to national titles, with Stewart leading undefeated teams in both 2013-14 and 2015-16.
We will be using WAR extensively in our college basketball coverage and highlight key players leading up to and during the NCAA Tournament. We can also look at conference-specific WAR metrics to better assess players within their league context and, yes, add DRIP for college basketball players.
We’ll also extend WAR historically – Caitlin Clark vs. Caitlin Clark is fun, but what about Caitlin Clark vs. Steph Curry?
Check out our NBA, NFL, college basketball, FBS and FCS coverage. And follow along on Instagram, Bluesky, Threads, Facebook and X for more.
This post was originally published on this site be sure to check out more of their content.